The idea behind Future Data Cringe was simple. I asked 50 global data leaders: What are we doing today as data teams that we’ll look back on in 20 years as cringe?
The responses came from current and former CEOs of companies shaping the data industry such as Snowflake, Tableau, and dbt Labs along with data practitioners who live and breathe analytics from companies like Wiz, Figma, and Meta.
What started as a brand initiative for Euno turned into a fascinating reflection on the current state of data teams. Though playful on the surface, Future Data Cringe took a hard look at the core practices data professionals take for granted today. One of the most upvoted quotes raised a bold question: Do we even need data teams in the future?
The theme of “cringe” made for some fun, but it also revealed recurring patterns that suggest major changes could be on the horizon for data teams. Here are five surprising themes that have surfaced from the Future Data Cringe collection that highlight the evolving nature of data work, and what might be cringeworthy in the near future.
1. Do We Really Need a Data Team?
The idea that a central data team that specializes in “data” and handles all analytics needs of the organization might be a thing of the past has been one of the most popular (and controversial) responses.
Many of the leaders discussed the potential for data democratization, where anyone in the organization could manage and analyze data without relying on a dedicated data team. Future data capabilities will spread across the business, much like a product management mindset is now needed everywhere.
These voices suggest that the future might lean toward more autonomous data systems, where tools and technologies empower non-engineers or non-analysts to perform the roles of traditional data teams. The question raised is whether we’re overcomplicating things by keeping teams siloed, and how AI might fill in the gaps for analysis and decision-making.
As your data operation scales (and with AI analytics in the mix) no central data team alone can keep up unless it adopts processes and tools that improve collaboration with business teams and empower everyone to contribute to the evolution of analytics.
Notable Quotes:
Jenna Jordan (#4) Ruth Onyekwe (#7) Eva Schreyer (#17) Richard Cotton (#18) Timo Dechau (#37) and Senne Vermassen (#43).
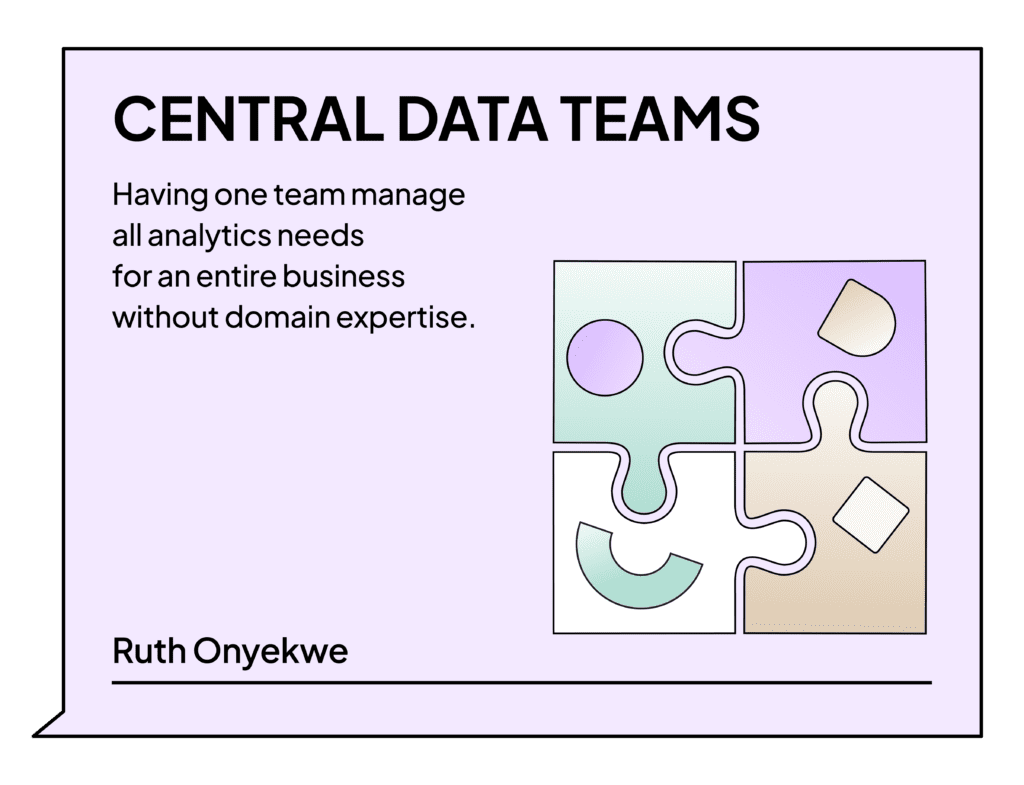
2. Too Much Manual Work
Another theme that surfaced was the overwhelming amount of manual work still involved in data management. From platform migrations to merging PR requests, many data leaders voiced frustration about how much of their time is spent on repetitive, non-strategic tasks. They’re wondering if automation and AI will eventually take over these mundane tasks to free up time for more creative and impactful work.
One perspective that stood out is how complex the modern data stack has become—often spanning 15-20 tools, from data warehouses and platforms to catalogs, semantic layers, BI tools, and visualization platforms. The immediate impact? Silos and conflicts caused by this artificial fragmentation. The long-term consequence? A growing pile of tech debt we’ve yet to fully reckon with.
Another interesting take came from ChatGPT itself. When asked what today’s data teams do that will surely make our future selves cringe, it called out how every minor transformation gets its own model—because data engineers hesitate to edit anything upstream. No one wants to slow down the business, yet no one has the time to manage business logic built by analysts. The result? A bloated tangle of dbt models.
Notable Quotes:
Tristan Handy (#1) Ross Helenius (#12) Lindsay Murphy (#15) Barr Moses (#19) Doron Porat (#22) Silja Märdla (#29) Kaitlyn Henry (#36) Matthaus Krzykowski (#38) Yair Weinberger (#40) Andrew Jones (#42) Jeremy Chia (#45) Jovita Tam (#47) Rachelle Calip (#49) and ChatGPT (#50).
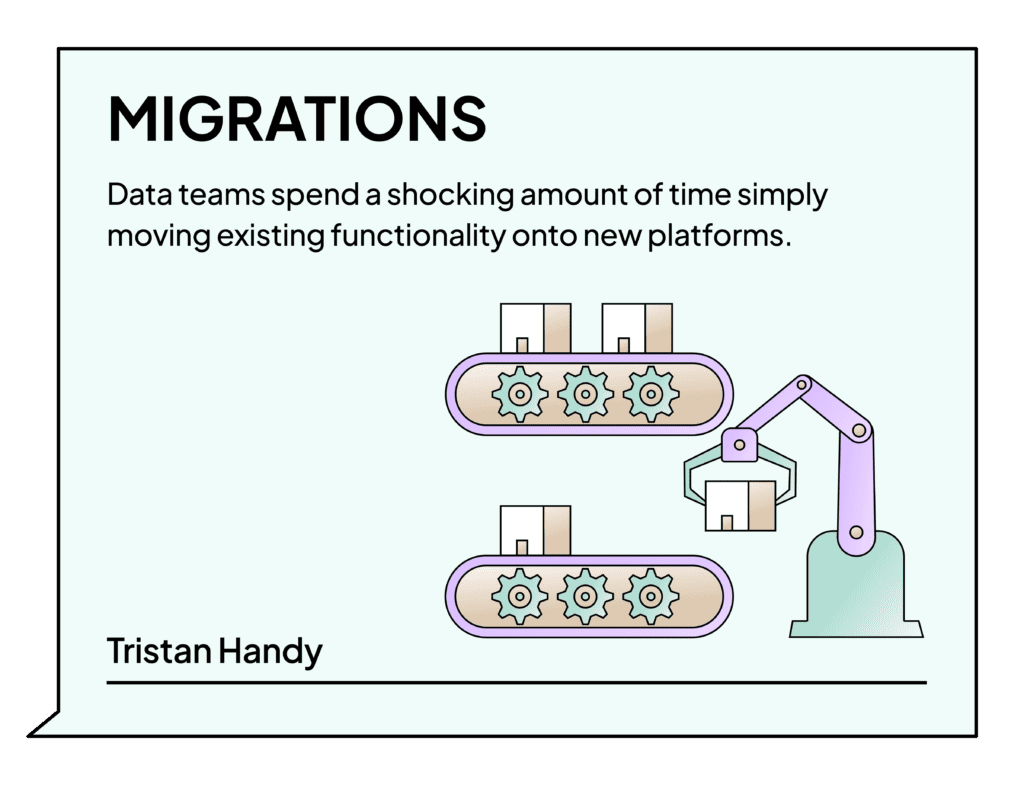
3. AI to the Rescue… or So They Say
AI was mentioned as a central figure in the potential evolution of data teams. The notion that AI could replace or drastically reduce the need for human intervention in many aspects of data science and analytics seemed to be a recurring thought.
One thing most data leaders cringed at was the idea that AI will replace analysts. As far as they’re concerned, analysts (and the craft of analysis) are here to stay. What will change is how we write queries. Most agreed that AI will take over that part for us.
Many of these experts emphasized that while AI isn’t perfect yet, its rapid advancements will likely drive fundamental shifts in how data engineering is approached. The real question is whether we’re preparing ourselves to fully integrate AI or whether we’re still holding on to outdated practices that AI could easily replace.
Notable Quotes:
Carlin Eng (#2) Bob Muglia (#5) Assaf Levinson (#9) Sarah Levy (#11) Richard Makara (#16) Tom Abraham (#25) Sharath Chandra (#28) Dan-ya Shwartz (#33) Omar Brid (#34) and Anurag Gangal (#41).
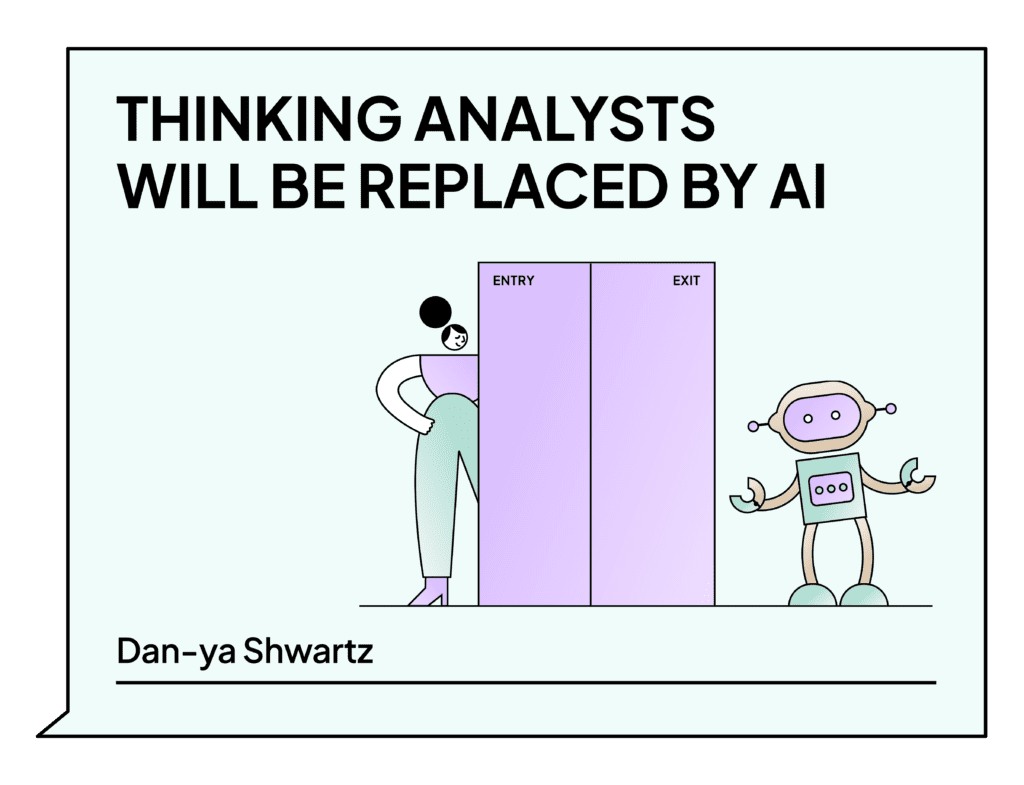
4. Farewell, Dashboards
We’ve all been there, building endless dashboards that ultimately aren’t used by anyone. The excessive focus on creating detailed, static visualizations that don’t serve a clear purpose was called out by many data leaders as something they anticipate future data professionals will cringe at. Rather than investing in sophisticated dashboards, there’s a growing argument that the focus should shift toward more actionable insights delivered directly to users.
The idea is that as AI evolves, users might bypass the dashboards in favor of more real-time, decision-driving insights.
Notable Quotes:
Kate Strachnyi (#3) Mark T. Nelson (#10) Nir Smilga (#20) Tatsiana Maskalevich (#23) Tim Hiebenthal (#30) Shenhav Lavie (#39) and Eyal Firstenberg (#48).
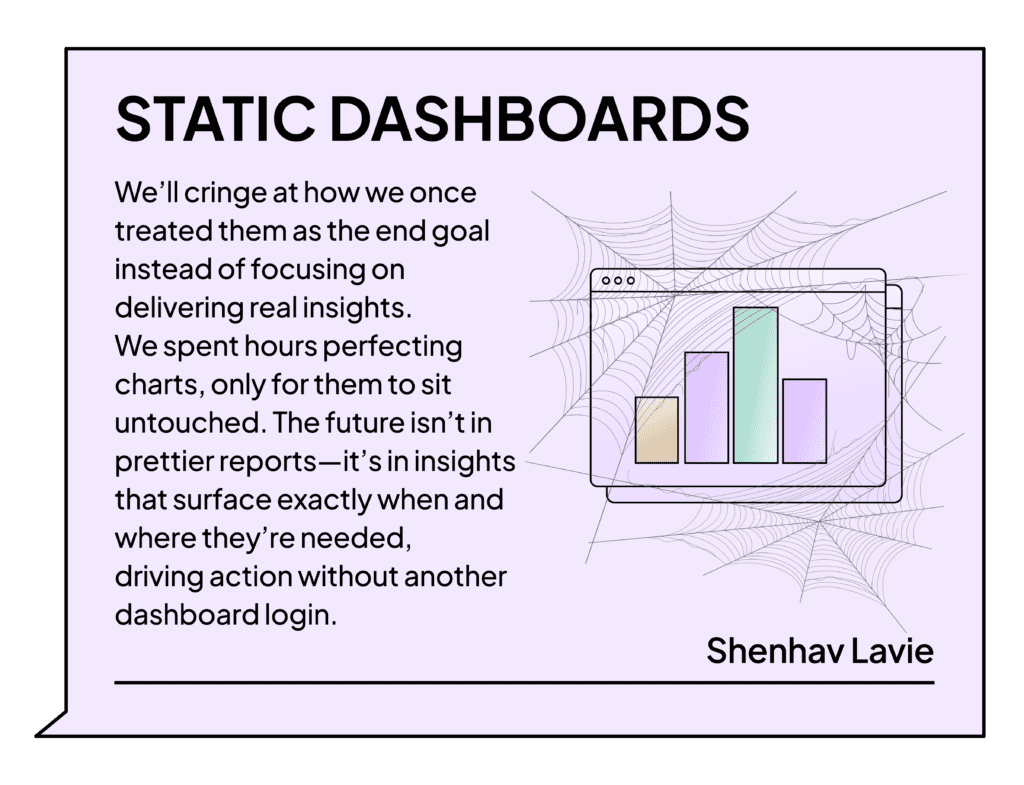
5. Habits We’ve Built but Should Rethink
Finally, several quotes called out the habits we’ve grown a little too comfortable with as data professionals. Whether it’s passive-aggressive table naming, data contracts over Slack, or deprioritizing data modeling → data leaders wished for a more proactive data team, one that leans into understanding the business and takes a more confident role in analytics and AI adoption.
These leaders suggest that future data teams might look back at today’s conventional habits and see them as barriers to efficiency, innovation, and scalability. The focus will likely shift to more flexible ways of managing data, and the old ways of doing things could quickly be left behind.
Notable Quotes:
Ravit Jain (#6) Tiankai Feng (#8) Gio Granato (#13) Lidor Avitan (#14) Ankur Batra (#21) Harry Gollop (#24) Joe Reis (#26) Dylan Anderson (#27) Benn Stancil (#31) Madison Schott (#32) Inna Weiner (#35) and Juan Manuel Perafan (#46).
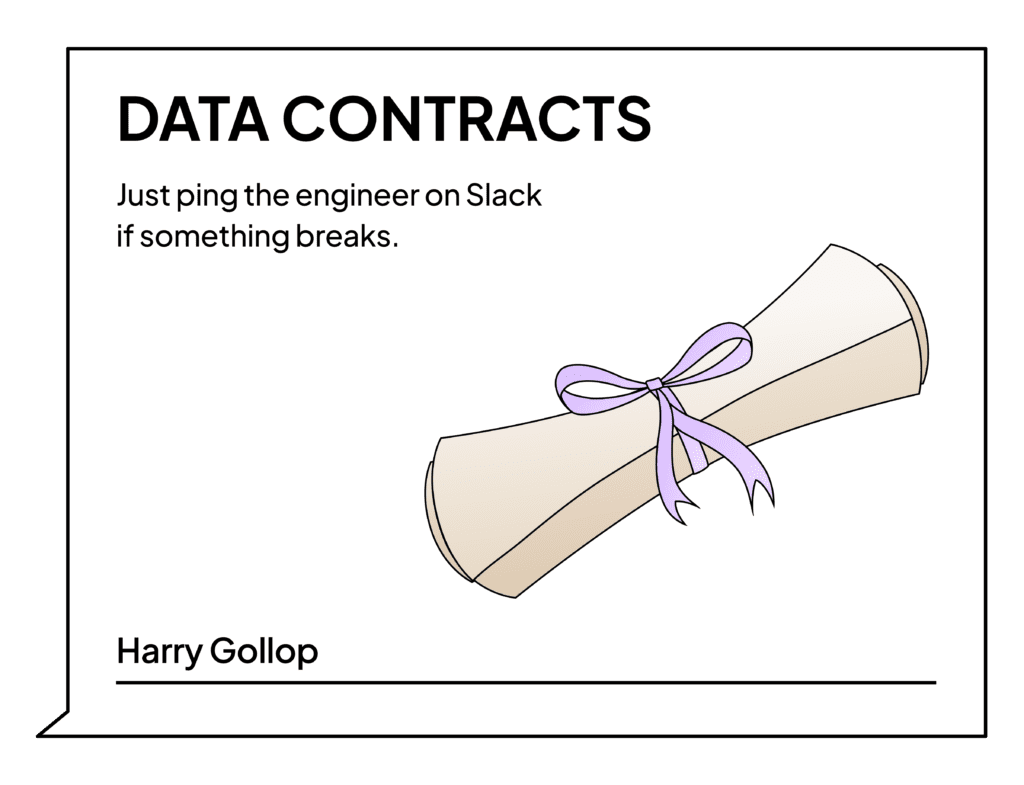
Euno’s Future Data Cringe: What You Can Do!
As self-service analytics becomes the norm, uncontrolled democratized data can lead to clutter, rising costs, and trust issues. With more people building data products and AI applications, the line between trusted assets and exploratory work often blurs, complicating AI deployment. To regain control, organizations need governance initiatives focused on migration management, cost optimization, data asset cleanup, metric alignment, and calculation discovery across teams.
Euno tackles these challenges through flexible governance powered by deep metadata. It automatically collects and connects metadata across layers capturing usage, lineage, and dependencies. Euno structures your metadata by turning code, comments, and descriptions into filterable, actionable insights. This enables data teams to understand the full context of their data assets, making it easier to manage and trust. Euno also activates governance with initiatives like decluttering, cost control, data asset certification, and metric standardization. This allows you to scale self-serve analytics without giving up your control over costs or governance standards.
Wrapping Up
It’s clear that the future of data teams will be shaped by AI, which already changes how we think about data. These recurring themes from the Future Data Cringe project highlight both the risks and opportunities ahead for data professionals. What seems normal today, even dashboards or the very existence of data teams, might seem downright cringe in the not–too-distant future.
While future data cringe looks lighthearted, it touches on basic issues relating to how data teams work today. By reflecting on these insights, data leaders can start to rethink how they operate today to avoid being on the wrong side of data history tomorrow.
Wait! How would you answer?